Effective AI training with LIDAR data
Artificial intelligence (AI) can only operate as powerfully as the quality of the trained data allows. In particular, this applies to deep learning, which uses neural networks inspired by the human brain. Tools for labeling camera images are already established on the market, but corresponding tools for labeling laser scanner data are not yet available.
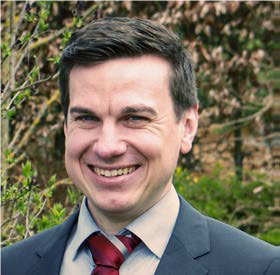
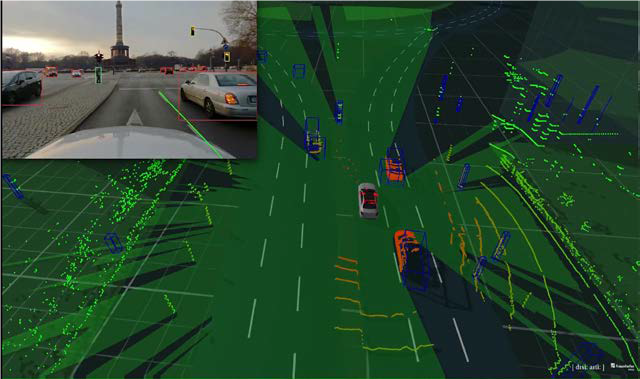
Mr. Schäufele, how can the labeling tool FLLT.AI pave the way to autonomous mobility?
In many self-driving vehicles, LIDAR sensors, laser scanners, are part of the sensor technology that is used for environment detection. To ensure that the vehicles can make any use of the data, the AI in the vehicle must be trained. For LIDAR data, however, there are only a few data sets available so far. With our labeling tool FLLT.AI, one can easily create datasets for LIDAR and simultaneously use recorded video data to automatically pre-label the LIDAR data. Furthermore it is easy to manually post-process the LIDAR data. In addition, tracking labels are used to continue from one LIDAR scan to the next. Thus, on average, only 10 % of the time is needed to generate high-quality learning data.
How do humans drive AI and will this work (almost) without them in the future?
Today, it is often the case that the AI needs to get specifications in a so-called “supervised learning” environment. Here, the data used for training must be processed by humans beforehand, labeling various objects in the data. In the future “unsupervised learning” may work without humans. In this case, the AI tries to determine differences in the data during the training and thus will be able to determine classes itself. However, checking whether these classes are correct and the determination of the parameters during trainings still has to be executed by a human.
How do microelectronics interact with your tool?
Through various interfaces offered by the FLLT.AI tool, data recorded with LIDAR sensors and cameras can be directly integrated into the FLLT.AI tool. We are also working on integrating other sensors, such as RADAR. For the data labeling, we use our own very powerful AI server farm to run the neural networks. At the same time, we are also working on the AI-Flex project to develop an ASIC specifically for running neural networks.
What is still missing for the autonomous vehicle that can be put on the road without worries?
Research into self-driving vehicles is already very advanced in terms of environment recognition. This means that traffic areas with relatively low complexity, such as highways, can already be mastered well. A major challenge is the decision-making in complex traffic scenarios, such as those found in cities, but also on rural roads with many intersections. The number of possible traffic situations there is very high and therefore requires a huge amount of data to prepare the AI.
Bernd Schäufele heads the Perception and Communication group in the Smart Mobility business unit at Fraunhofer FOKUS. He has participated in large Europe-wide research projects in the field of vehicle communication and cooperative driving, such asSimTD, Drive C2X and TEAM. His research focuses on cooperative positioning and cooperative driving maneuvers as well as environment recognition with LIDAR and camera sensor technology. In Bernd Schäufele’s group, the software framework “FLLT. AI” was developed for the automatic and manual labeling of LIDAR data and for the fast generation of highquality data for artificial intelligence training. Bernd Schäufele holds a master’s degree in software systems engineering from the Hasso Plattner Institute in Potsdam.
Last modified: