Reliable microelectronics through failure analysis with artificial intelligence
The use of machine learning methods offers novel possibilities for automating and thus increasing the efficiency of failure diagnostics. Together with partners, the Fraunhofer IMWS wants to pave the way for this in an international project. The new methods based on artificial intelligence (AI) will help to capture and evaluate complex failure modes.
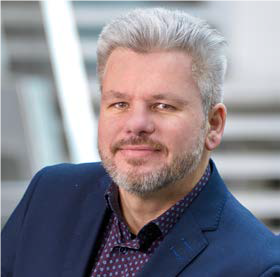
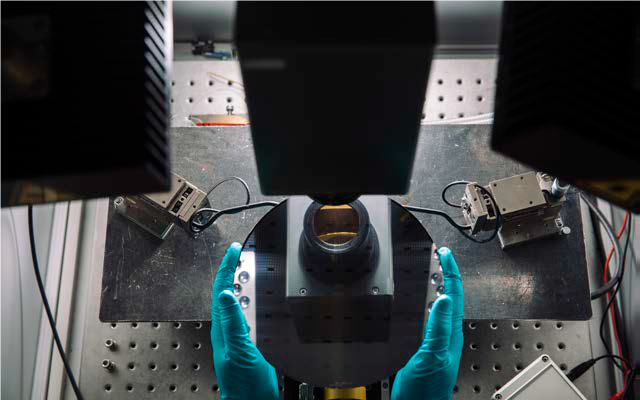
This is precisely where the project “Failure Analysis 4.0 – Key for reliable electronic devices in smart mobility and industry production” (FA 4.0) comes in. Until March 2023, the partners from research and industry are working on methods for failure analysis tools and workflows. “High integration density is a constant challenge for the quality and reliability of components for microelectronics,” says Frank Altmann, who leads the activities of the Fraunhofer Institute for Microstructure of Materials and Systems IMWS within the new project. “Powerful and continuously improved methods for failure analysis are therefore elementary.”
Largely automated error analysis and data management
To achieve this common goal in the project, Fraunhofer IMWS focuses on the research and development of AI methods for signal analysis of non-destructive defect detection– for example acoustic microscopy or lockin thermography. The idea: to develop concepts of supervised as well as unsupervised machine learning and then integrate them into diagnostic devices for data acquisition and evaluation. On the one hand, the researchers are working on linking individual analytical devices from different stages of the process with standardized hardware and software interfaces and, on the other hand, on defining guidelines and database systems for structuring, processing and storing component-specific characteristic and analysis data. Once a link between data from different analysis methods and the layout of an integrated circuit has been achieved and subsequently analyzed by a trained and self-learning algorithm, defects could be automatically detected, their signature determined, classified and compared with catalogued data on already known causes of defects. These new innovative approaches can identify previously undetectable complex defect patterns and significantly reduce the time and cost of defect analyses.
Successful fault diagnostics with AI-based evaluation
Frank Altmann is optimistic about the future and sums up the project: “In current failure analysis workflows, numerous methods are used that provide complementary analysis data from components. However, these have so far only been linked manually. The automated, cross-process provision and AI-based evaluation of this data, including derivation of failure causes, have enormous potential to further increase the efficiency of quality control in manufacturing and thus increase product reliability and quality with ever shorter development times.”
Frank Altmann has been acting head of the Business Unit “Materials and Devices for Electronics” at the Fraunhofer IMWS in Halle (Saale) since 2019. In addition, he has headed the group “Diagnostics Semiconductor Technologies” since 2006 and has been lecturing at Merseburg University of Applied Sciences in the master’s program “Mechatronics, Industrial Engineering, Physical Engineering” since 2007. He himself studied physics at the TU Dresden and already wrote his diploma thesis in cooperation with the Fraunhofer Institute in Halle. He has been working at the Fraunhofer IMWS since 1997 and most recently conducted research on fault diagnostics for 3D integrated microelectronics.
Last modified: